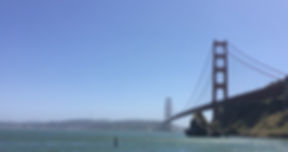
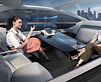
A Deep Dive into the AV Ecosystem
March 2019
Among the megatrends currently revolutionizing the mobility space, autonomous driving is both the most transformative and probably the most R&D intensive endeavor. Tech giants and startups have forced incumbent players into a race to market autonomous vehicles (AVs), aiming to reduce road casualties, offer mobility to more people and enable better use of time.
Huge efforts are being undertaken to enable this revolution, with a short-term focus on shared mobility applications, whether for people or for goods. These efforts are evidenced by the development of a broad and dense ecosystem. It is comprised of tech giants, startups and established OEMs and Tier 1 suppliers which develop the hardware or software solutions necessary to bring AVs to market. What are the key building blocks of this ecosystem? What are the dynamics of the various segments? Let’s start with the extensive landscape I have co-produced with ReadWrite Labs (hi-def version here).
Structure of the AV Landscape
The AV ecosystem includes players involved in hardware (HW), software (SW), vehicle and fleet applications, and analytics layers. The AV Landscape (above) contains about 300 companies. It is not exhaustive, but is updated quarterly — this is the third iteration. OEMs have been omitted as they are all engaged at some level in the development of AV solutions. In order to better understand the ecosystem, I added a second level to the underlying taxonomy. Let’s drill down into the various segments.
Autonomous Vehicle Hardware
The “AV Hardware” segment gathers companies that provide sensors (lidar, cameras, radars, etc.), compute solutions, on-board communication products and V2X solutions. The enabling technologies include compute platforms with higher processing power and at lower cost, AI chips, new sensor tech and high capacity / low latency communication solutions.
The lidar (LIght Detection And Ranging) space has recently attracted a lot of investment and talent. First developed for military applications, lidar are considered a must-have for SAE Level 3 (eyes off) and above, except by Tesla. A lidar is a laser-based 3D scanning sensor which outputs a point cloud. There are two main technologies: spinning or solid state. Whereas the former type is available today and costs thousands of dollars, the latter is expected to be mature within a few years and to cost hundreds of dollars. Lidar performance is assessed against its point cloud density, its range for a given object reflectivity (the best claim 200 m at 10%) and its horizontal and vertical fields of view. Though Velodyne (cloud point image below) has had the most market presence since the DARPA challenge in 2004, Valeo is the first company to install a lidar on a production vehicle, the Audi A8.
Other sensors needed to operate autonomously include cameras, radars and ultrasonic sensors. Cameras are the only sensors in the AV suite that can read and recognize colors. There are therefore necessary to understand road signage and traffic signals. Cameras can also provide depth thanks to recent computer vision algorithms, and therefore generate a 3D rendering of a scene. Radars provide distance to an object and offer either a short range or a long range (over 250 m), with a shrinking field of view as the range increases. Lastly, ultrasonic sensors address the immediate proximity of a vehicle (up to 5 m) for maneuvering purposes.
Lidars, cameras and radars provide a 3D representation of the environment around a vehicle. They are to some extent redundant, which adds safety to the AV system. However, they do not all operate in all conditions, e.g., lidars can barely see through fog or rain unlike radars. Whereas Tesla intends to go to full autonomy with cameras and radars only, the rest of the industry adds lidars to their sensor suite. If cameras, radars and ultrasounds are mainly offered by incumbents, lidars are essentially developed by new players, though often integrated in the offering of Tier 1s.
The compute HW is the brains of the AV system which Intel predicts will generate about 4 TB of data per day. Recent developments rely on high performance GPUs and dedicated AI chips to provide the processing and neural networks necessary for the core AV tasks, i.e., perception, prediction, path planning and actuation. The most powerful platform today is Nvidia’s Pegasus with 320 TOPS of deep learning capability (see below). The company provides its core chips to established Tier 1s for integration on their boards. Unique among OEMs, Tesla is currently developing its own compute platform and announced significantly higher performance than existing solutions.

The V2X — or vehicle-to-everything — segment encompasses companies that provide solutions enabling a vehicle to communicate with other vehicles (V2V), the infrastructure (V2I), other devices (V2D), e.g., smartphones, or the cloud (V2C). Most AV system developers do not want to rely on real time V2X to operate their drivers for reliability and latency reasons. However, V2X make it possible to see another vehicle around a corner or to detect that a vehicle several cars ahead is suddenly braking. V2D will allow communications with pedestrians, i.e., possibly informing them of an AV’s intention. Two V2X technologies are in competition: one is cellular-based (C-V2X) and the other WiFi-based (DSRC and ITS-G5).
Given the massive amount of data generated by the multiple sensors and the need for the lowest latency possible, CAN buses are being replaced by Ethernet for onboard communication. A few companies have developed the required cyber secure gateways and switches, such as CetraC.
Autonomous Vehicle Software
The “AV Software” category contains companies that develop the AV SW stack for light vehicles or trucks, localization and mapping solutions, simulation and validation tools and contents, as well as development tools.

Companies developing full stack AV SW are by and large startups, some being very well funded such as Aurora Innovation which recently raised $530M. A few tech giants are also investing heavily, such as Waymo, the leader in this space (developing SW and HW stack), Uber, Lyft, Baidu or Apple (in stop and go mode). Lastly, a handful of OEMs and Tier 1 suppliers have gained strong positions throughout the acquisition of startups, such as GM with Cruise (now valued around $15B), Ford with Argo AI or Delphi with nuTonomy. Others are developing the tech in house, such as Daimler, Renault-Nissan, Audi (AID) or Valeo.
AV SW stack developers for trucking applications are different, with the exception of Waymo and Uber. The most mature player here is probably Sino-American TuSimple, valued around $1B.
A critical component of an AV solution is localization and mapping. AV-specific 3D, high-def, annotated maps are created to provide an understanding of the scene where a vehicle operates, e.g., lanes, curbs, signs, and enable precise localization (see below by Civil Maps). Various companies develop proprietary maps as no standards have been defined. Some players enable real time updates via a crowdsourcing approach in order to account for abnormal events, e.g., construction sites.

Simulation is a growing space, as it enables AV developers to test SW versions and HW configurations in multiple scenarios, with quick iterations. Simulation is necessarily combined with real life tests. Whereas a few major AV developers have built their own simulator, e.g., Waymo with Carcraft (about 15B simulated km to date), most AV developers use off-the-shelf simulators and banks of annotated data sets to test their models. Even human behavior can be simulated, thanks to Latent Logic.
Lastly, a few companies provide services to enable AV development. Solutions include data annotation, programing tools, camera tuning with Algolux, or the supply and installation of AV HW with Autonomous Stuff.
Autonomous Vehicle Applications
“AV Applications” relate to companies that deploy AVs or provide services targeting AV fleets. Though AVs are only taking baby steps, new players have emerged to manage fleets, addressing vehicle dispatch, health monitoring or traveller interface such as BestMile. A few players like Phantom Auto have recently emerged with the objective to operate AVs remotely, i.e., to get AVs out of difficult situations without having to dispatch an operator.
Several startups have emerged to build — and sometime operate — vehicles that autonomously transport people or goods. The earliest companies on the passenger side were 2getthere, EasyMile and Navya. The latter two each operate close to 100 autonomous shuttles with no steering wheels or pedals (Level 4, though with programmed waypoints). The best funded player is Zoox (about $800M raised to-date); the Silicon Valley-based company intends not only to design and build robotaxis, but also to operate a ride-hailing service.

But the most advanced AV operator is Waymo, which started to run the Waymo One ride-hailing service in Phoenix last December. A fleet of Chrysler Pacificas fitted with Waymo’s proprietary AV HW and SW operate at Level 4, though back-up drivers remain onboard for the time being.
Things are moving fast as well for the transport of goods, especially the last mile delivery. Vehicles will operate at low speed (up to 40 km/h), either on the street or on sidewalks. Nuro, which recently raised $940M, appears to be the most advanced player in this space. The various players are already conducting multiple pilots across the globe with such vehicles.
Analytics
I focused here on data analytics layers related to a vehicle’s occupants. “Driving aid and monitoring” is not directly linked to AVs, as these companies focus on providing a safety layer for human-driven vehicles. Their solutions assist drivers in their task, based on the understanding of the driving scene. A by-product can be a reduction in insurance premiums, using driving score derived from behavior analysis. Nauto is probably the most mature player in this space.

Lastly, a segment that has been so far largely untapped is the understanding of what goes on inside the cabin. Generally camera-based, solutions output a range of features from driver’s awareness, eye gaze, emotions, age, sex, race, head pose all the way to body pose, occupant actions and forgotten objects for Eyeris, the most mature player (see above). This information will increase safety as well as enable new services.
Conclusion
It will it be years before technology can provide a level of safety that surpasses the 1 road traffic death per 100M vehicle-kilometers human drivers achieve today across all sorts of road, traffic and weather conditions. Nevertheless, the deployment of AVs has begun in specific operational design domains (ODD). These ODDs will only grow larger with time. As the tech matures, so will the ecosystem. Whereas some of the the startups we see today will grow as independent players in their own right, more will be absorbed — many have received investments from incumbents. Alternatively, their business with OEMs will be channeled through Tier 1 suppliers. Unfortunately, the majority will disappear. For the time being, the AV ecosystem will remain vibrant.
Finally, I wish to thank Kailash Suresh and the rest of the ReadWrite Labs team for the productive collaboration that made this AV Landscape possible.
Marc Amblard
Managing Director, Orsay Consulting
Feel free to comment or like this article on LinkedIn. Thanks!